EP 2 789 960 B1 relates to a method for determining the condition of a fire-resistant lining of a metallurgical melting vessel.
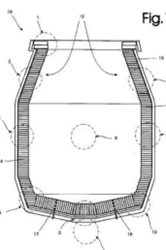
The claimed method used measured or established data of each vessel which are stored in a data structure, whereby a calculation model is generated from at least some of the measured or ascertained data or parameters.
Brief outline of the case
The OD revoked the patent for lack of sufficiency of all requests, cf. Art 83.
The board confirmed the revocation.
We will concentrate on the MR in appeal.
The proprietor’s point of view on sufficiency
The sophisticated “computational model” underlying the invention is a machine learning model.
In view of the rapid progress made in recent years in the field of machine learning, the creation of a suitable computational model of machine learning, unlike at the time of filing the application on which decision T 161/18 was based, is now a matter of common general knowledge and does not require an express disclosure.
All the variables mentioned in the claim and their relevance to wear are known to the skilled person in the field of steel production.
The efficiency of the claimed invention results, on the one hand, from the fact that the parameters relevant to wear are mentioned and, on the other hand, from the nature of machine learning, in which the predictive power is acquired by self-learning without prior knowledge of the analytical relationships.
The proprietor further argued that that a skilled person in the field of data analysis would recognise from the term ‘adapted’ that it must be a self-adapting ‘adaptive model’ and thus a machine learning model.
Moreover, the large number of input variables also points to machine learning, since an analytical approximation formula is not applicable to such a multidimensional problem.
Finally, claim 5 according to the MR discloses with the expression ‘the model for this neural network’ that the computational model in claim 1 refers to an artificial neural network. Consequently, the skilled person understands from the overall context of the invention in the patent that claim 1 relates to a method of machine learning.
For the proprietor, precise knowledge of the relationships and the respective influence of the individual parameters on wear, on the other hand, is not necessary for sufficiently because it is precisely the essence of machine learning that the ability to predict the output variable without knowledge of the causal relationships is acquired by self-learning through training. The influence of irrelevant input variables is filtered out automatically.
A ‘research programme’ would only be necessary if the causal relationships and the significance of each input variable were to be clarified. This effort is not necessary for a machine learning model.
The opponent’s point of view on sufficiency
The invention according to claim 1 is not limited to machine learning and is therefore not practicable due to the lack of information on the computational model.
The patent does not contain a single concretely reworkable embodiment example and no more detailed information on the choice of parameters, the concrete representation of the parameters in the computational model, the choice of the computational model of machine learning to be created and how the required training data can be obtained.
The disclosure in the patent in suit was therefore even less specific than in the case of the invention found to be unworkable in decision T 161/18.
The board’s decision on sufficiency
The term ‘computational model’ is not restricted to a model from the field of machine learning in connection with an adaptation. The fact that the model is ‘adapted’ is not synonymous with the model being ‘adaptive’, i.e. self-adapting or self-learning.
A regression analysis can be used both to adapt the coefficients of an analytical approximation formula and to adapt the weights in an artificial neural network. The large number of input parameters therefore also does not mean that the calculation model must be a machine learning model.
The patent provides neither an example of such a computational model nor indications of the relationships to be modelled, so that the disclosure of the patent does not enable a skilled person to set up such a model and to carry out the invention in this field of the claimed subject-matter.
In this respect alone, the case in question differs significantly from that dealt with in decision T 161/18 discussed by the parties, in which at least the neural network used was specified in the underlying application. In said decision, the sufficiency was denied solely due to a lack of information on suitable input data for training the neural network.
In the present patent, it is even left to the skilled person alone to determine a calculation model suitable for the required task.
The general technical knowledge put forward by the proprietor may provide the skilled person with some guidance as to which architectures and types of computational models are better or less suitable for which problems in general but are not related to the specific task of the present invention.
It is therefore left to the skilled person to find out what is important for the calculation model according to the invention, without the patent or the general specialised knowledge providing any assistance.
Even assuming that claim 1 is limited to a machine learning that the assignment of the data to input and output variables and the calculation of the wear is comprehensible to the skilled person it would not be possible without unreasonable effort on the basis of the available specialised knowledge to select a calculation model that is also suitable for the specific problem.
The patent does not contain any information or a single example of which specific measured values could be selected or are particularly meaningful for wear. For each of these partially mixed-value, time-dependent or possibly vectorial, multi-parametric or multi-dimensional variables, the skilled person must define a representation that can be processed for the calculation model, without the description comprising any, at least exemplary, teaching.
The lack of detail of disclosure in the patent is disproportionate to the breadth of the claimed invention and the corresponding effort for a person skilled in the art to fill in the gaps in order to be able to carry out the invention.
Comments
It has to be agreed with the board that if the invention in T 161/18, is not sufficiency disclosed, this is even more the case here.
What the proprietor claims is that the skilled person knows what matters when deciding upon the wear of a metallurgic vessel, and once the necessary data are inputted, the computational model will learn by itself in order to obtain the desired result. Claim 1 of the MR defines no more than a result to be achieved.
Not only training data are missing in the present disclosure, but the skilled person is left completely in the dark as to how the correlation of the input data leads to a output allowing to decide on the wear of the metallurgic vessel. Simply claiming that a neuronal network can be set up to obtain the desired result is by far insufficient as on top of it no hint about the training data to be used.
That neuronal networks can be used to determine wear and tear of a metallurgic vessel is known in the field, but it needs a bit more information to come to a sufficient disclosure.
It is as if, in the field of autonomous driving, an applicant would give a set of rules for motor management, road circulation rules, driving patterns and digital road maps to determine a route with the minimum wear and tear for the car and that a neuronal network would, with some magical wand, give the expected result.
Comments
Leave a comment